「BAGSS Internal Methods Institute 2025」
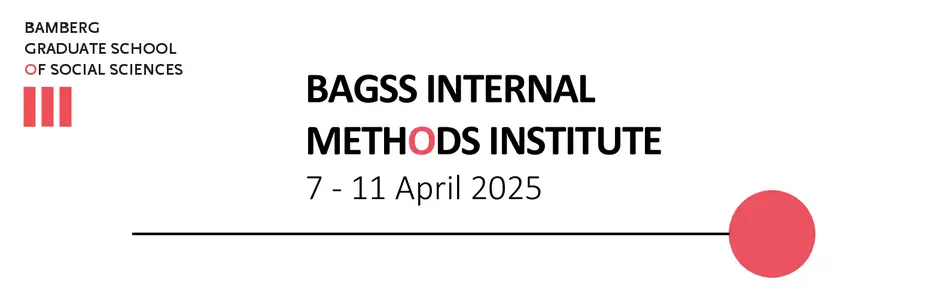
「INTRODUCTION」
Welcome to the 4th BAGSS Internal Methods Institute! Our methods institute is designed to provide you with a week long of comprehensive training, equipping you with skills you need in your research. Besides expanding your skill set in empirical social research, we are also happy to invite you to the side events which will accompany and enrich the experience.
「COURSES」
Case-Based Research Design
INSTRUCTOR: Professor Dr Derek Beach, Aarhus University
Instructor
Derek Beach is a professor of Political Science at Aarhus University, Denmark, where he does research on research methodology and European integration. He has authored articles, chapters, and books on research methodology, policy evaluation, international negotiations, referendums, and European integration, and co-authored the book Process-tracing Methods: Foundations and Guidelines (2019, 2nd edition, University of Michigan Press). He has taught case study methods at numerous workshops and ph.d. level courses throughout the world, and conducted evaluations at the national and international level. He was an academic fellow at the World Bank’s Independent Evaluation Group in spring 2022 and is an academic coordinator of the Methods Excellence Network (MethodsNet).
Course outline
This five-day course provides a hands-on introduction to case-based designs, enabling participants to use them in their own research. The course is designed to be relevant for participants from a range of different social science disciplines. A constant theme throughout the course will be on debating the strengths and limitations of different case-based methods, illustrating the types and scopes of inferences that are possible, and how they differ from what variance-based methods enable.
+ More Information(307.3 KB)
.......................................................................................
DATE & TIME: 07.04 - 11.04.2025
PLACE: FG1/FMA
Timetable
Monday
will first introduce the foundations of case-based designs in terms of taking cases as the analytical point of departure. The course will distinguish between comparative and within-case designs.
Tuesday
deals with concepts and theories in case-based designs. After discussing how concept structure is dealt with, we will explore different types of theoretical relationships that are assessed using case-based designs, including necessary and sufficiency, as well as theories of causal processes (aka mechanisms).
Wednesday
unpacks different tools for comparative analysis, including more simple comparisons using tools such as Mill’s methods, and most-similar and most-different systems designs. Participants will also be introduced to the basics of Qualitative Comparative Analysis (QCA).
Thursday
deals with within-case analysis, focusing in particular on process-tracing methods. Using a published example, we will discuss how theories about causal processes can be developed and what elements they should include, as well as how process tracing works with empirical evidence.
Friday
presents the most central case selection principles, including typicality, deviant cases, and theoretical likelihood (most- and least-likely cases). We will then discuss how cross-case comparisons and within-case tracing can be combined in practice.
Machine Learning for Macroeconomic Modeling
INSTRUCTOR: Tato Khundadze, The New School for Social Research
Instructor
Tato Khundadze is a political economist currently working as a Teaching and Research Assistant at the New School for Social Research in New York City, where he is pursuing his PhD in Economics. Additionally, he holds the position of Associate Professor of Economics at the Georgian American University in Tbilisi, where he teaches intensive courses in statistical analysis. His research interests include development economics, trade policy and industrial policy.
Course outline
This course aims to provide participants with an in-depth understanding of advanced modeling and control techniques applicable to economic systems. Through a combination of theoretical knowledge and practical case studies, students will explore Nonlinear Model Predictive Control (NMPC), machine learning, and dynamical systems identification. The course will also highlight the application of these concepts to urgent global challenges, including climate change and wealth disparities.
+ More Information(184.7 KB)
.......................................................................................
DATE & TIME: 07 - 11 April 2025, 9.30 - 11.00 a.m. & 11.30 a.m. - 1.00 p.m.
PLACE: FG1/FMA, Feldkirchenstra?e 21, 96052 Bamberg
Timetable
Lecture 1
- Nonlinear Model Predictive Control (NMPC) and the Implementation of the NMPC Package in Python
Lecture 2
- Climate Models with NMPC in Python
Lecture 3
- Unsupervised Learning – Case Study: Monetary Policy and the Evolution of Wealth Disparities
Lecture 4
- Introduction to Deep Learning – Case Study: A Deep Learning Approach to Analysing Conspicuous Consumption Patterns Among U.S. Consumers
Lecture 5
- Deep Reinforcement Learning and Its Application to Macroeconomics – Case Study: Using Reinforcement Learning and NMPC to Model Cooperative Fiscal Policy in the Euro Area
Lecture 6
- An Introduction to Sparse Identification of Nonlinear Dynamical Systems (SINDy) and its Application in Macroeconomics
Multilevel Modeling
INSTRUCTOR: Research Professor Levente Littvay, HUN-REN Centre for Social Sciences
Instructor
Levente Littvay is a Research Professor at HUN-REN Centre for Social Sciences and Senior Research Fellow at the Democracy Institute of Central European University, where he also was (Full) Professor of Political Science and taught graduate courses in research design, applied statistics, electoral politics, voting behavior, political psychology, and American politics and was the inaugural and only two-time recipient of the university's Teaching Award. He received his MA and PhD in Political Science and an MS in Survey Research and Methodology from the University of Nebraska-Lincoln, taught numerous research methods workshops globally, and is the founder and Academic Coordinator of MethodsNET, a Presidium member of the Hungarian Political Science Association, and head of Team Survey in Team Populism. He was a member of the European Social Survey’s Round 10 democracy and COVID-19 module questionnaire design teams and Co-Principal Investigator for the Comparative Study of Election Sys-tems for Hungary and Tunisia. His awards include the European University Institute’s Fernand Braudel Senior Research Fellowship, and the 2022 Giovanni Sartori Prize for best paper in the Italian Political Science Review, and the Morton Deutsch Award for the best 2017 article in Social Justice Research. His books include Multilevel Structural Equation Modeling with Bruno Castanho Silva and Constantin Manuel Bosancianu in SAGE’s QASS (little green book) series, which was also published in Mandarin Chinese.
Course outline
Multilevel data structures are all over the social sciences: observations within municipalities, cantons, districts, or countries. Students within schools. Patients within hospitals. Multiple observations taken from the same person or any other unit of analysis. In quantitative studies, relevant predictors appear on all these levels of analysis. But how do we deal with them?
The course is designed to provide scholars with a basic understanding of multilevel (a.k.a. Hierar-chical linear or mixed effects) regression models designed to solve these problems. Special attention is given to the translation of theoretical expectations into statistical models, the interpretation of results in multilevel analyses, and the general use and misuse of multilevel models in the social sciences. While the course is predominantly designed to give you the knowledge of multilevel regres-sion modeling, it does also arm you with the basic tools to run multilevel models in R. (I also have Stata code for most of the models presented thanks to a former teaching assistant, but I am not a Stata user so there you are on your own.) Applications will include models with continuous and limited dependent variables in hierarchical, longitudinal, and cross-classified nesting situations and, if time allows, multilevel structural equation models. The goal of the course is to offer a basic intro-duction and the foundation for participants to start using and critically assessing multilevel models and also have the ability to independently discover and master advanced multilevel statistical topics. Upon completion, the participants will have a basic conceptual understanding of multilevel modeling and its statistical foundations. Participants will be able to critically assess the appropriateness of such techniques in their own and other people’s research and conduct multilevel modeling them-selves to the highest academic standards.
+ More Information(159.3 KB)
.......................................................................................
DATE & TIME: 07 - 11 April 2025, 9.30 - 11.00 a.m. & 11.30 a.m. - 1.00 p.m.
PLACE: FG1/FMA, Feldkirchenstra?e 21, 96052 Bamberg
Prerequisites
A solid foundation in linear regression. (Knowing what to click in SPSS and how to copy and paste the table does not constitute a solid foundation. Knowing the assumptions of regression models like homoskedasticity and no autocorrelation does.)
The Statistics of Causal Inference
INSTRUCTOR: Professor Dr Elias Dinas, European University Institute
Instructor
Elias Dinas holds the Swiss Chair in Federalism, Democracy and International Governance. He holds a PhD in Political Science from the European University Institute (2010) and his research interests include the dynamics of political socialization, the downstream effects of institutional interventions and the legacy of authoritarian rule on the ideological predispositions of citizens in new democracies. He has also a keen interest in research methodology. His work has been published, among others, in the American Political Science Review, the American Journal of Political Science, the Journal of Politics, and Political Analysis and mentioned in The Economist, the Atlantic and the New York Times.
Course outline
The objective of this workshop is to learn how statistical methods can help us to draw causal claims about phenomena of interest. Participants will be introduced into an authoritative framework of causal inference in social sciences, i.e. the potential outcomes framework. By the end of the course, students will be in position to:
1. critically read and evaluate statements about causal relationships based on some analysis of data;
2. apply a variety of design-based easy-to-implement methods that will help them draw causal inferences in their own research.
3. think about archival data under the logic of causal inference. Either explicitly or implicitly, the goal of most empirical research is to interpret causally the co-occurrence of interesting phenomena.
Addressing causality, however, has been notoriously difficult without the luxury of experimental data. This course will introduce you to methods that allow you to make convincing causal claims without working with experimental data. In the first part of the course, we will look at three such designs:
1. Difference-in-Differences estimation;
2. Instrumental Variables and;
3. Regression Discontinuity Design
For every method, the following structure will be employed: first, a running example will provide the motivation and intuition. We will then proceed with the formal identification derivation and finally we will focus on estimation strategies and robustness checks. For each method there will be a hands-on lab section, where we will apply these methods with real data.
We will not have the time to cover two topics, which I would love to talk about: attrition and bounds.
Do ask me about this towards the second part of the course.
+ More Information(196.9 KB)
.......................................................................................
DATE & TIME: 07 - 11 April 2025, 9.30 - 11.00 a.m. & 11.30 a.m. - 1.00 p.m.
PLACE: FG1/FMA, Feldkirchenstra?e 21, 96052 Bamberg
Timetable
Monday
- Introduction to the Potential Outcomes Framework
- Motivation, examples, discussion. We will see examples of the fundamental problem of causal inference. Introduction to the potential outcomes framework. We will derive the causal quantities of interest.
- How experiments solve the fundamental problem of causal inference. The logic of randomization and why it works.
Tuesday
- Randomization given by nature: Examples of Natural Experiments
- Instrumental Variables: Intuition, Identification & Estimation, Wald Estimator, 2SLS Estimator
Wednesday
- Regression Discontinuity Design: Motivation, Identification, Estimation Strategies.
- Testing for Sorting, Robustness Checks, Examples, Applications.
Thursday
- The Fuzzy RD
- The Local Randomization Framework
- Extensions: Identification away from the Cut-off point & Randomization-Based Inference in the RD design.
- Lab Session: Regression Discontinuity Design
Friday
- Difference-In-Differences: Motivation, examples, identification, estimation.
- Threads to validity and examples; Gentle introduction into Staggered DiD
........................................................................................................................................................
MAIN PAGE | CONTACT | LEGAL | PRIVACY POLICY | DATENSCHUTZ | IMPRESSUM
? Bamberg Graduate School of Social Sciences